Data integration for large-scale models of species distributions
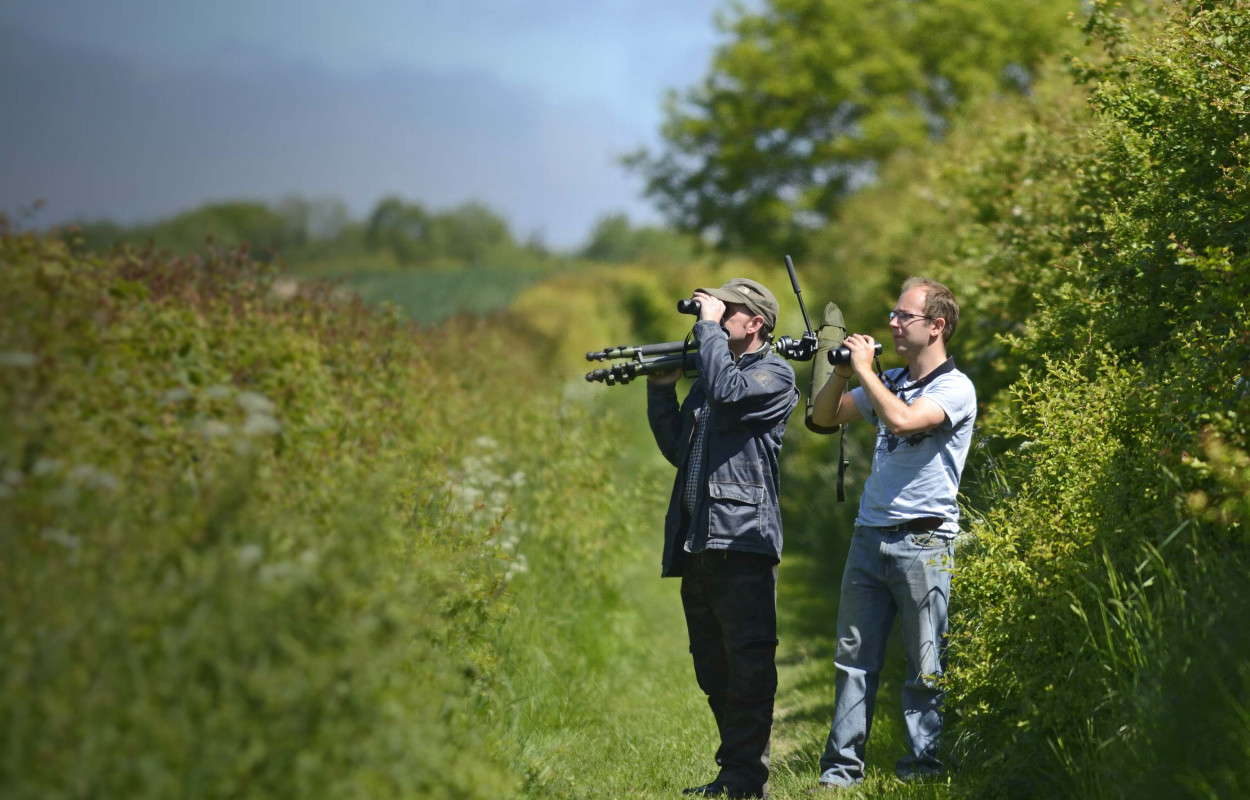
Author(s): Isaac, N.J.B., Jarzyna, M.A., Keil, P., Dambly, L.I., Boersch-Supan, P.H., Browning, E., Freeman, S.N., Golding, N., Guillera-Arroita, G., Henrys, P.A., Jarvis, S., Lahoz-Monfort, J., Pagel, J., Pescott, O.L., Schmucki, R., Simmonds, E.G. & O’Hara, R.B.
Published: October 2019 Pages: 12pp
Journal: Trends in Ecology & Evolution
Digital Identifier No. (DOI): 10.1016/j.tree.2019.08.006
Every year more and more people contribute records of the animals and plants they observe around them to citizen science schemes. The rapid growth of this form of biodiversity recording means that ecological data are being collected at an unprecedented rate and on vast spatial and temporal scales. For example, BTO’s BirdTrack scheme has received over 5 million records in the current year alone.
These massive data sets have the potential to radically improve our understanding of species distributions in time and space, and provide critical information for species monitoring and conservation planning. However, citizen science schemes differ in their format and scope, and consequently the type and quality of data collected. They span a broad spectrum from unstructured schemes, which allow the recording of single species observations at a time and place selected by the participant, to highly structured schemes which follow rigorous observation protocols at predefined survey locations, such as BTO/JNCC/RSPB Breeding Bird Survey. Combining data sets from different schemes can maximize the available information about species distributions and trends, but it is important to account for the properties of different data sources. For example, structured surveys tend to deliver very accurate and unbiased data, but are often limited in their coverage, whereas unstructured schemes may provide very large sample sizes but can suffer from numerous forms of bias, such as preferential sampling at locations with convenient access.
Traditional analytical methods tend to be tailored to a single data source, requiring analysts to choose among data sets. The published review highlights a novel analytical framework which enables the integration of different data sources into a single statistical model, retaining the strengths of each input. The modelling approach explicitly separates the biological and data generation processes and can be applied to a wide spectrum of data types, spanning haphazard observations and systematic population counts. It is based on so-called point processes, which are statistical descriptions of the way animals or their home range centres are distributed in space. The review features case studies demonstrating the application of this integrated modelling approach to citizen science data sets of trees, butterflies, frogs, and birds. While some questions remain open, in particular about how much structured data is required to overcome possible biases in unstructured data sets, the integrative approach opens a promising avenue of research, and holds the potential to gain a better understanding of the distribution of rare and uncommon species that are currently poorly covered by schemes such as the Breeding Bird Survey.
Abstract
Integrated modeling of species distributions and abundance is emerging as a powerful tool in statistical ecology.
Point processes provide a flexible framework for developing integrated models, combining data representing the locations of individual organisms, local population abundance, and species–site occupancy.
These methods provide opportunities to make best use of existing and new data sources.
We expect that data integration will underpin the next generation of models predicting the current, future, and potential distributions of species.
With the expansion in the quantity and types of biodiversity data being collected, there is a need to find ways to combine these different sources to provide cohesive summaries of species’ potential and realized distributions in space and time. Recently, model-based data integration has emerged as a means to achieve this by combining datasets in ways that retain the strengths of each. We describe a flexible approach to data integration using point process models, which provide a convenient way to translate across ecological currencies. We highlight recent examples of large-scale ecological models based on data integration and outline the conceptual and technical challenges and opportunities that arise.
Share this page